Compress it! 8bit Post-Training Model Quantization
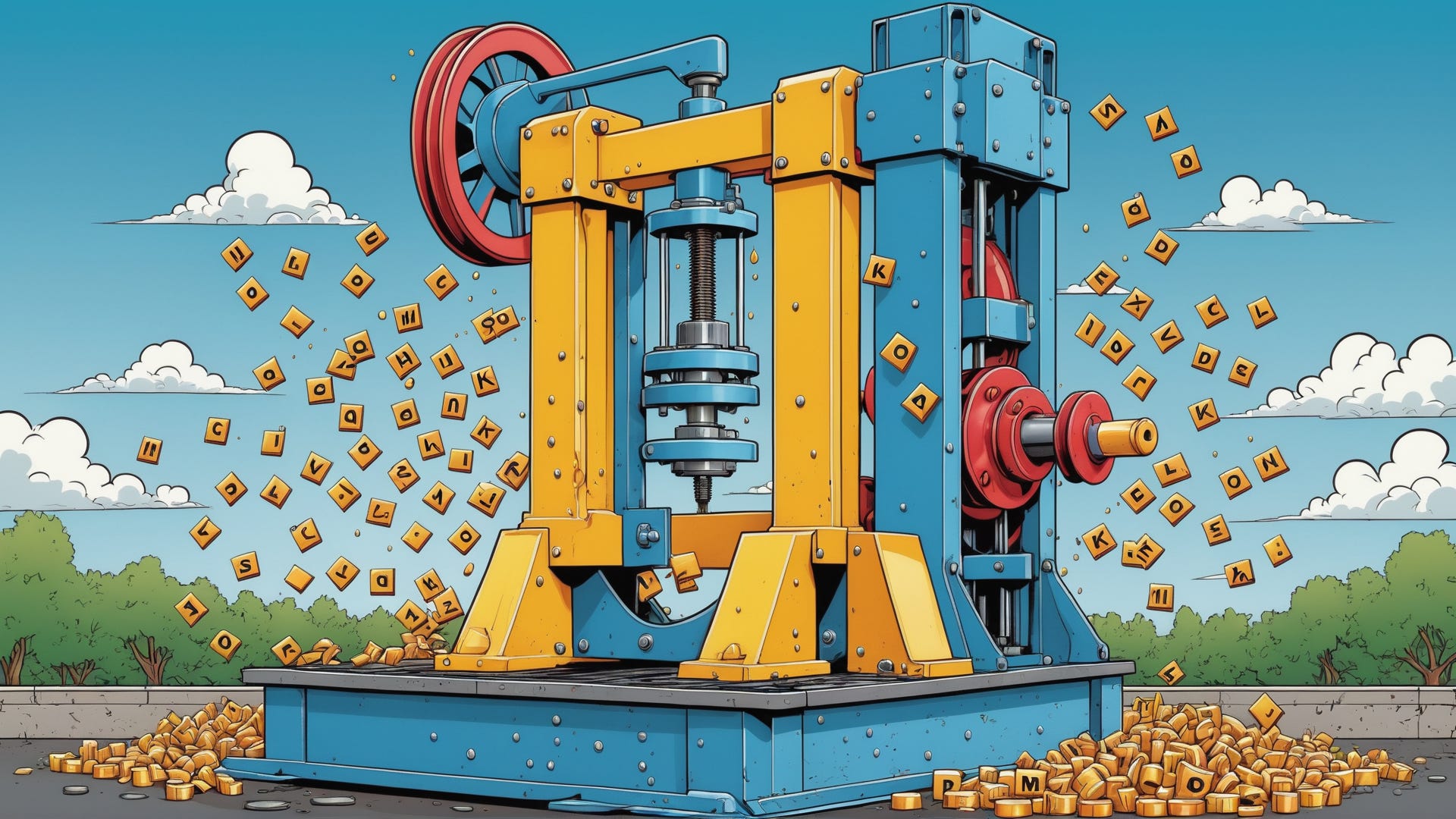
This week, I want to share with you a few notes about the 8bit quantization technique of a PyTorch model using Neural Network Compression Framework. The final goal is to squeeze the model to obtain excellent performances for a local inference on your device, without spending money on expensive new hardware or cloud API providers.
To achieve this goal we are going to navigate several different steps starting from the download of a PyTorch fine-tuned model, pre-trained on MRPC (the Microsoft Research Paraphrase Corpus).
Continue Reading >>>
A Ray of light in scaled Generative AI
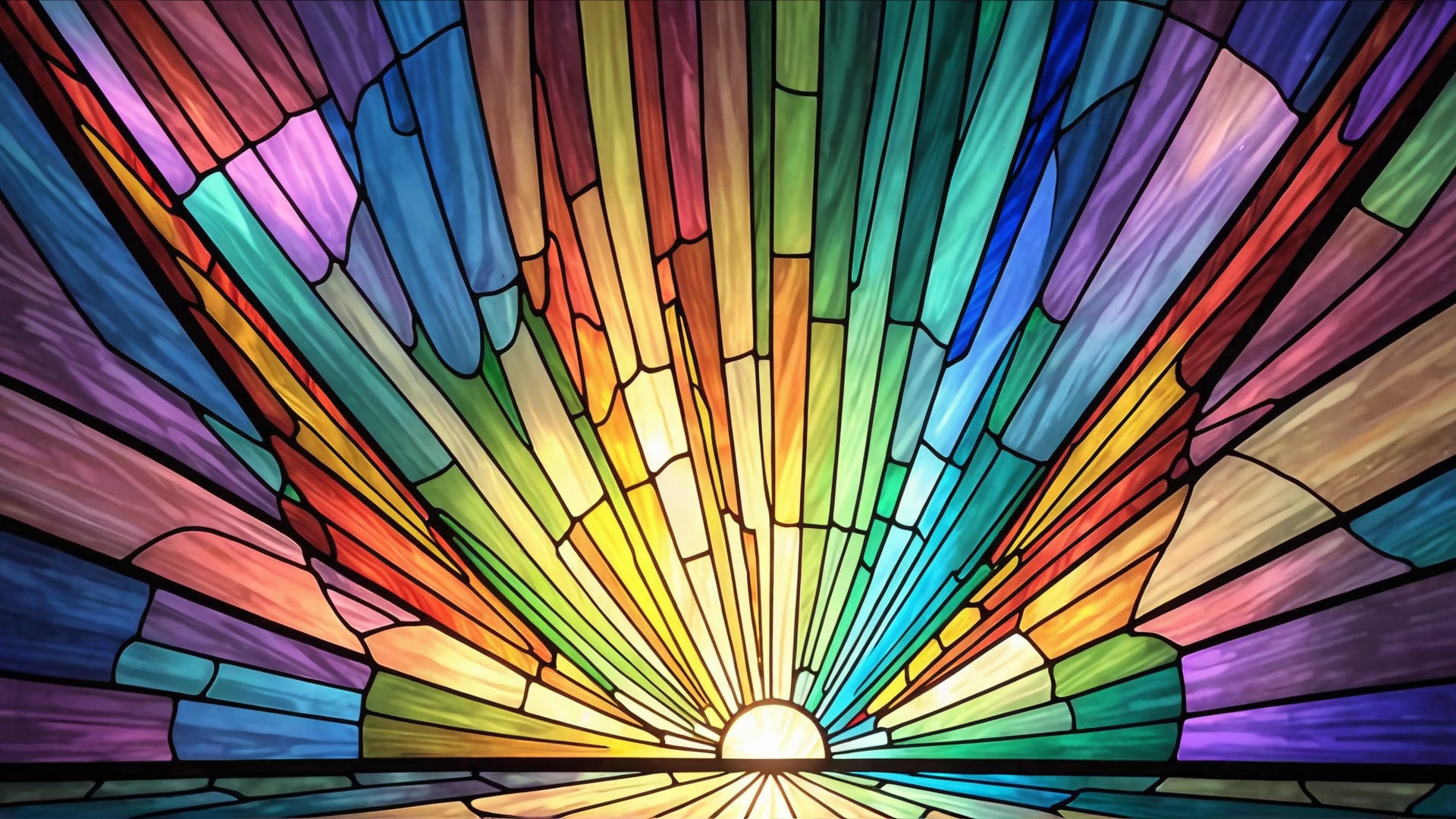
This week I would like to make a step forward in presenting the opportunity of running advanced local AI capabilities (like models fine-tuning, retrieval augmented generation, langchain-based applications, etc…) based on open source frameworks specifically designed for CPU.
After Neural-Speed, NeuralChat, auto-round, and Intel Extension for Transformers, it’s time to play with llm-on-ray,
Before embarking in the exploration of Intel/llm-on-ray, we need to take some time to discover the framework on which it is based: Ray.io by Anyscale.
Continue Reading >>>
Advanced Weight-only Quantization Technique on CPU
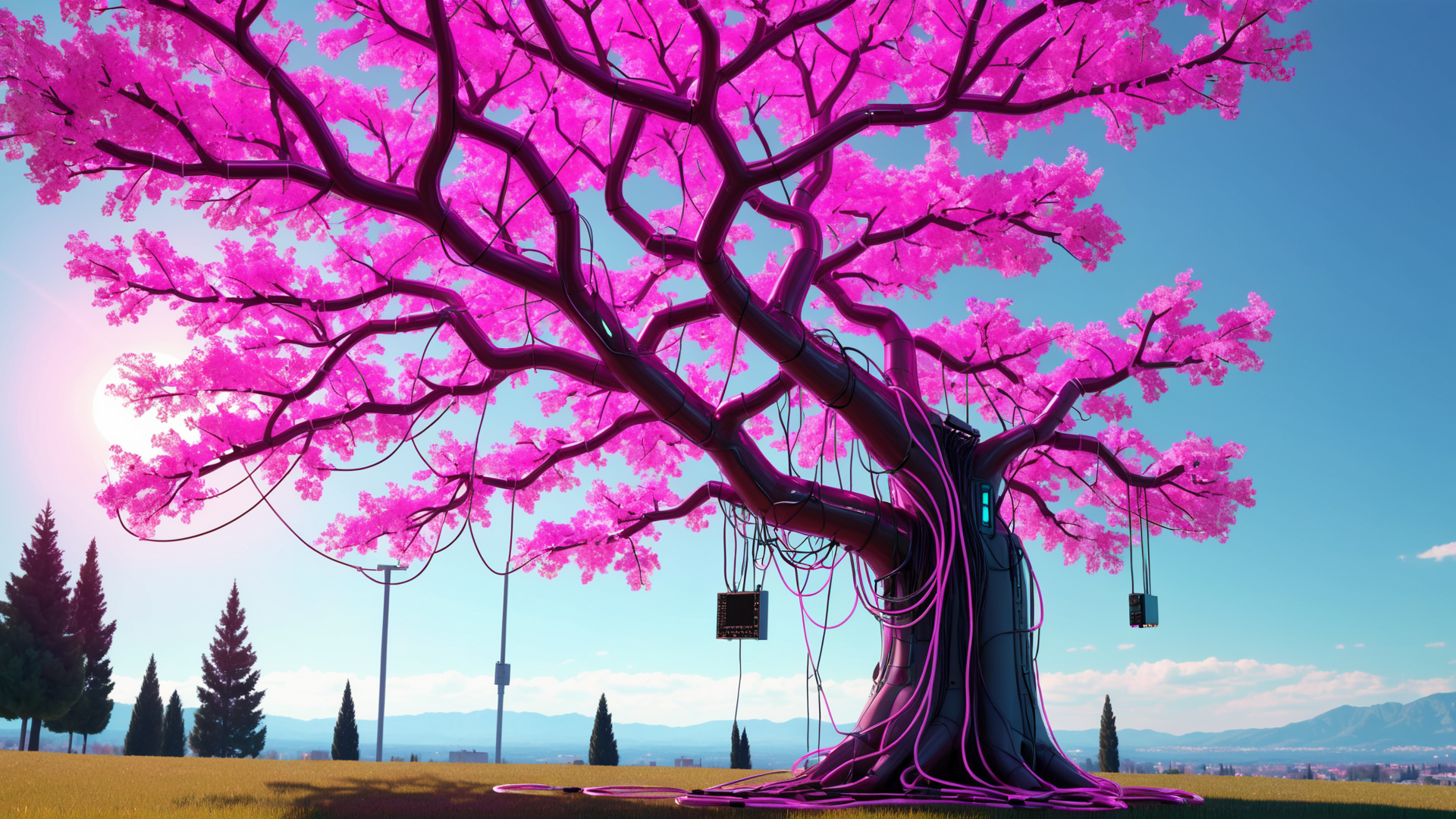
When LLMs started spreading at the end of 2022, it sounded something really impossible: training or even just fine-tune a model on your modest customer-grade hardware was fantasy.
Now, in the middle of 2024, thanks to an intensive work of scientific research, considerable investment, open governance, open collaboration, and a good dose of human ingenuity, we are now able to fine-tune models directly on our devices. Incredibile!
Continue Reading >>>
NeuralChat: deploy a local chatbot within minutes
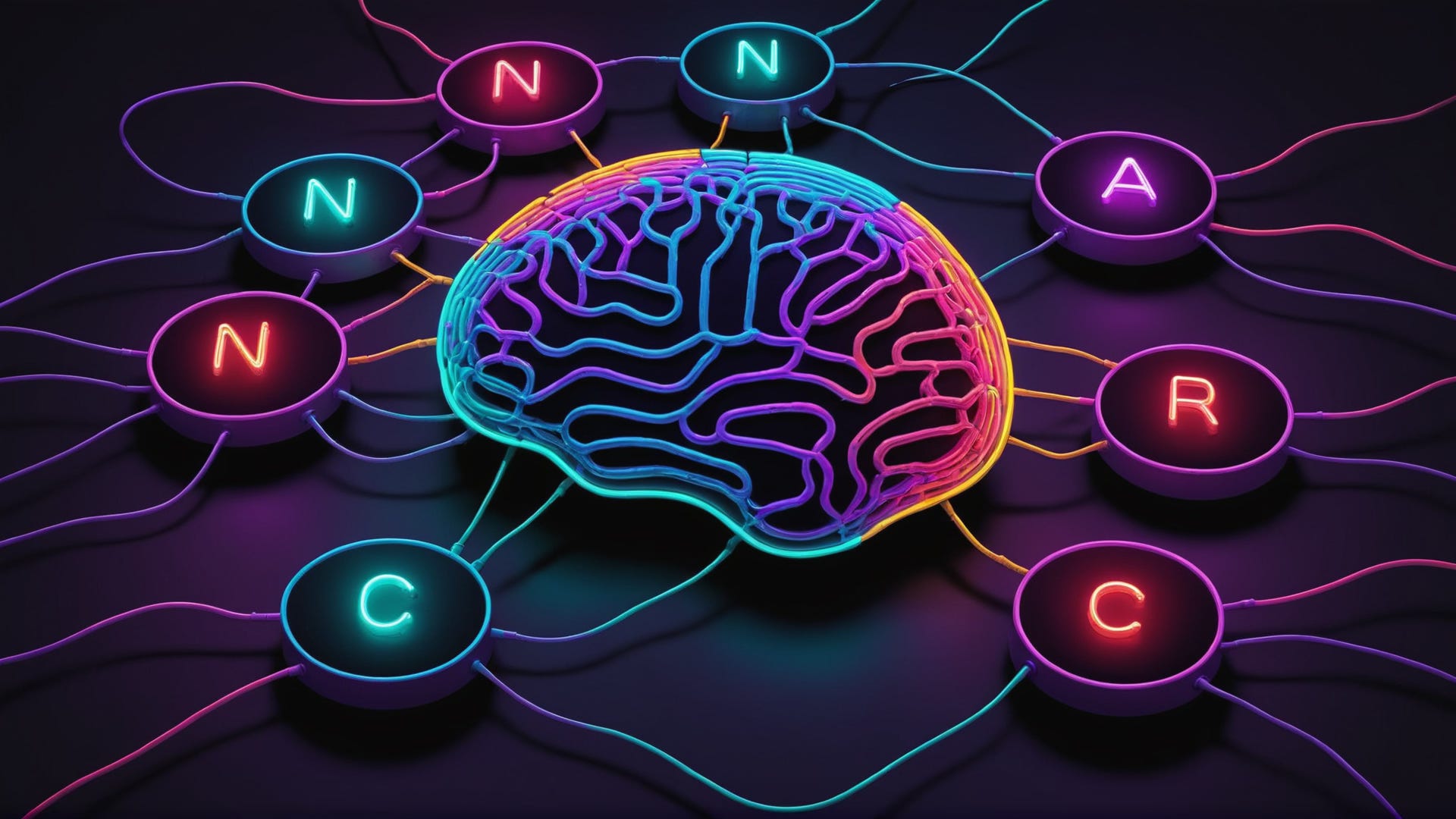
After showcasing Neural Speed in my past articles, my desire is to share a direct application of the theory: a tool developed using Neural Speed as very first brick, NeuralChat.
NeuralChat is highlighted as “A customizable framework to create your own LLM-driven AI apps within minutes”: it is available as part of the Intel® Extension for Transformers, a Transformer-based toolkit that makes possible to accelerate Generative AI/LLM inference both on CPU and GPU.
Continue Reading >>>
Neural Speed, Advanced Usage
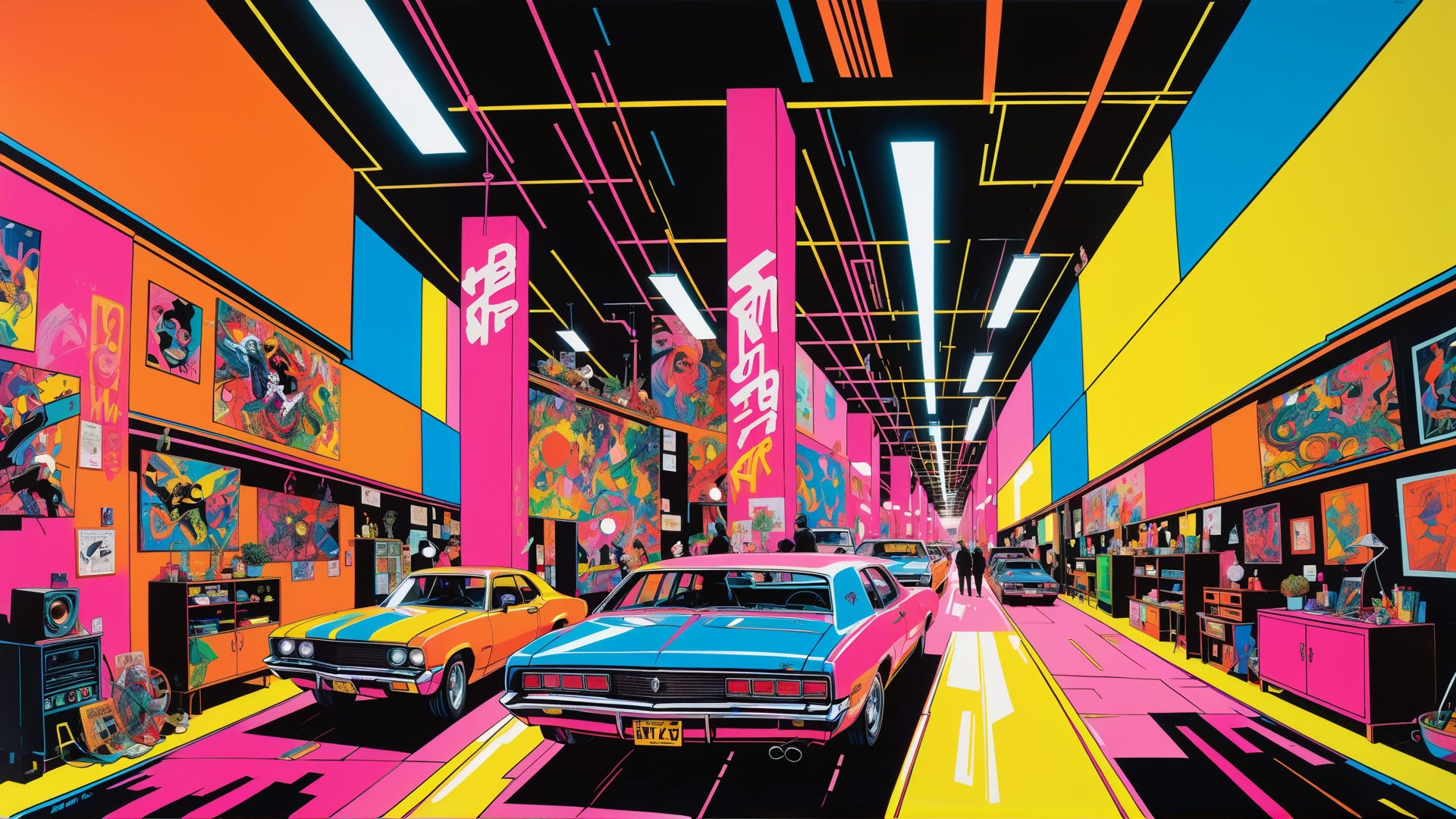
After an initial presentation and a second follow up, here the third episode of my excursus about weight-only quantization, SignRound technique and their code implementation: the tensor parallelism library and inference engine, Neural Speed.
It is an amazing tool, and makes sense to explore a little more all the opportunities it offers through its multiple options.
Continue Reading >>>
Efficient LLM inference on CPU: the approach explained
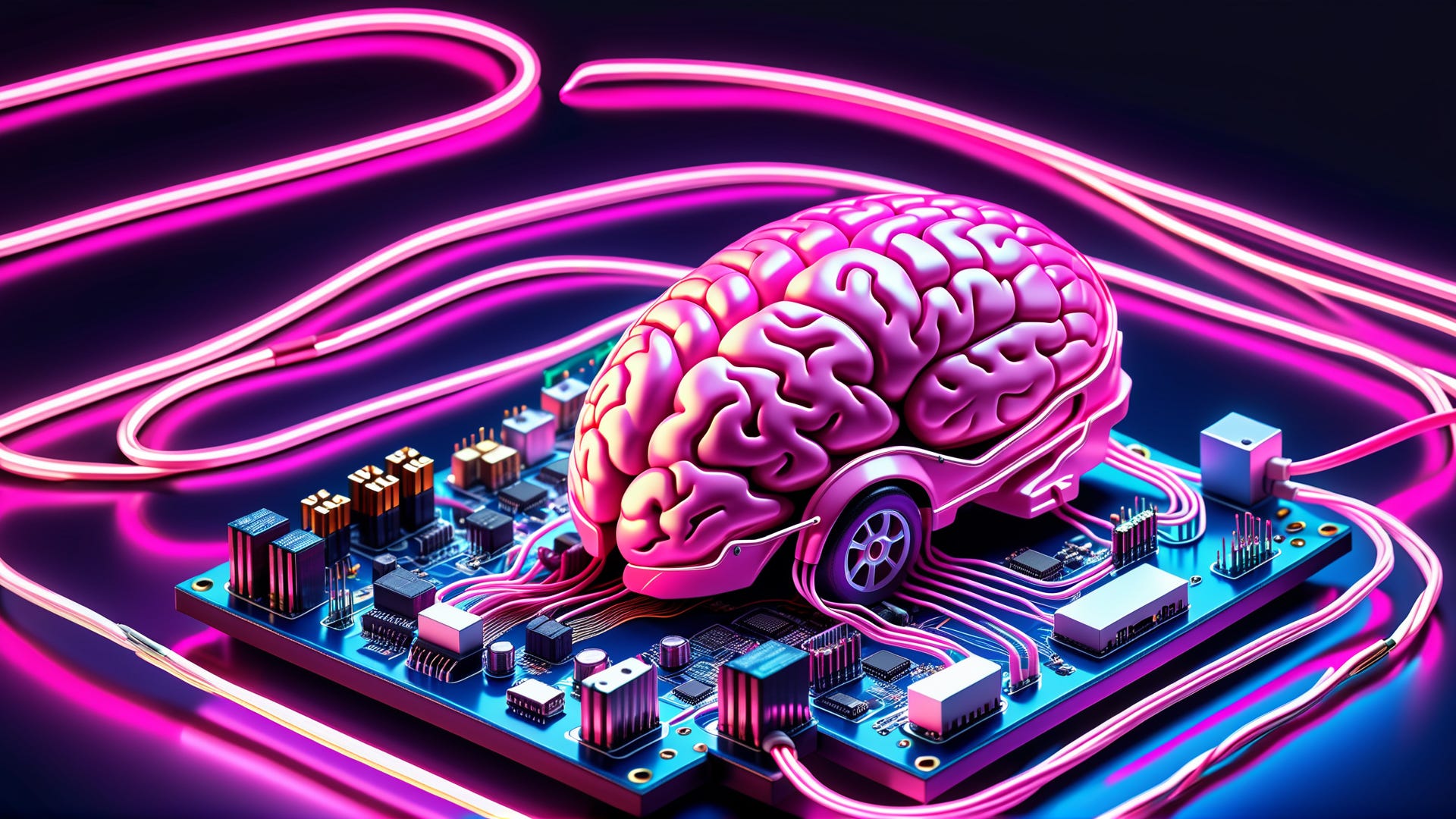
In the previous article I presented you a new inference engine, Neural Speed, that demonstrates incredible performance and that can run proficiently on consumer-grade CPU, without the need for expensive graphic cards or other dedicated resources.
Before proceeding in taking a deep dive into the advanced features of Neural Speed, it makes sense to take a seat and try to understand how it works under the hood. So, the intention of this article is to directly report a few concepts from the original documentation of Neural Speed.
Continue Reading >>>
Effectual LLM inference on Intel CPUs
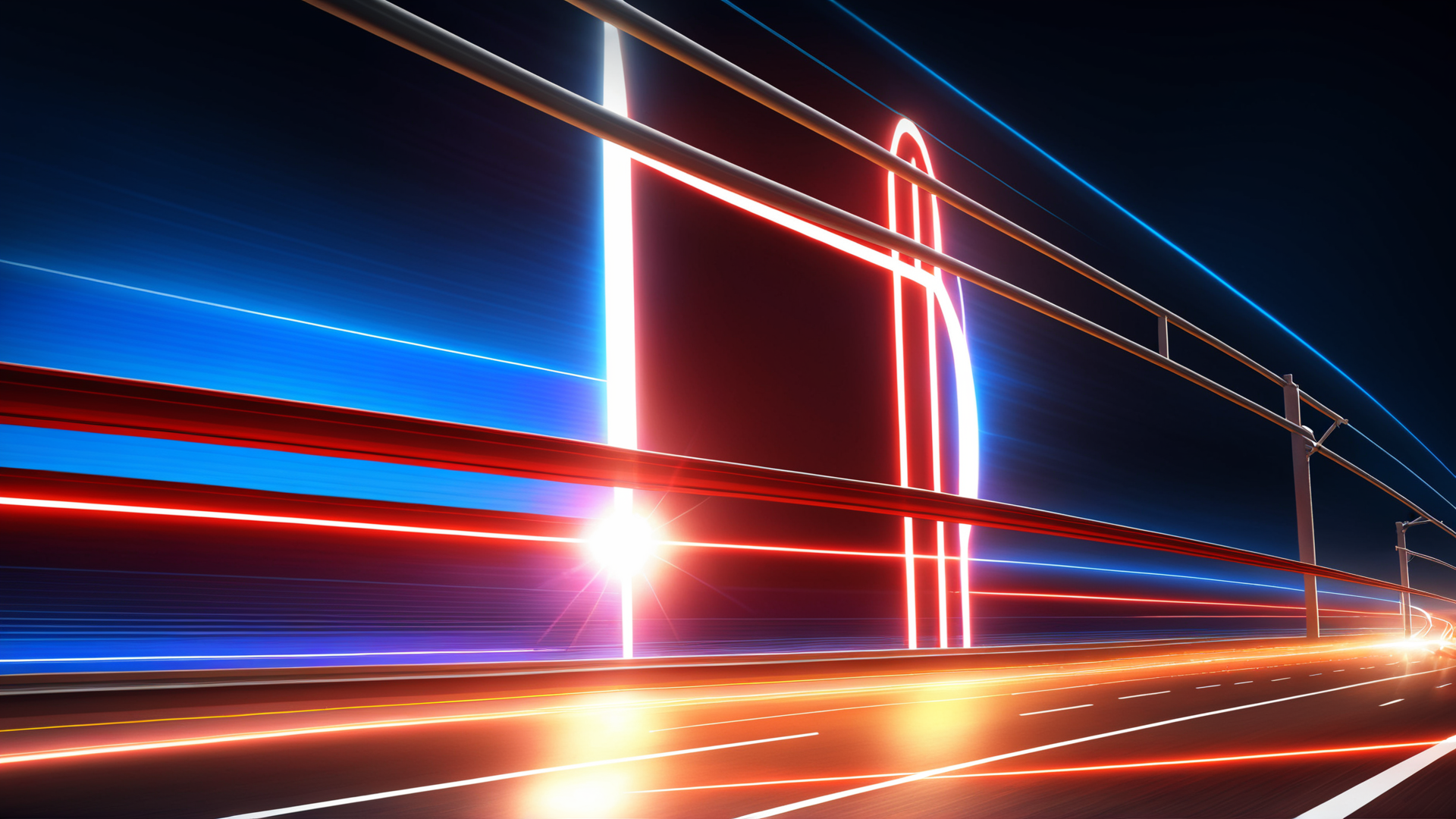
LLMs (Large Language Models) are incredible: they have demonstrated phenomenal performance and a proven potential across thousands of different scenarios. However, they require massive amount of computational resources to be executed: starting from basic elements like several gigabytes of storage, and astronomical quantity of GPU vRAM. Really impractical for many users who would like to execute simple natural language tasks, such as classification or entity recognition, and the only hardware available is usually consumer-grade. The more obvious solution is usually taking advantage of mainstream cloud providers (the triad Amazon AWS, Microsoft Azure, Google Cloud Platform), that make available services designed to provide direct APIs and offers, but the scarcity of GPU availability, the chip shortage, and the high demand raised the costs of this online platforms. Costs that are not affordable for the vast audience of software developers, data scientists and researchers, students, hobbyists, little professionals that want to try out solutions based on LLMs and build something innovative from scratch without the need to rely on third party services and continuous stable connection to Internet; not to mention the privacy and security of external providers that is not always fully guaranteed.
The alternative I want to propose is to develop solutions that could take full advantage of your personal hardware.
But, how is it possible to deploy large language models considering their immense amount of parameters?
Continue Reading >>>